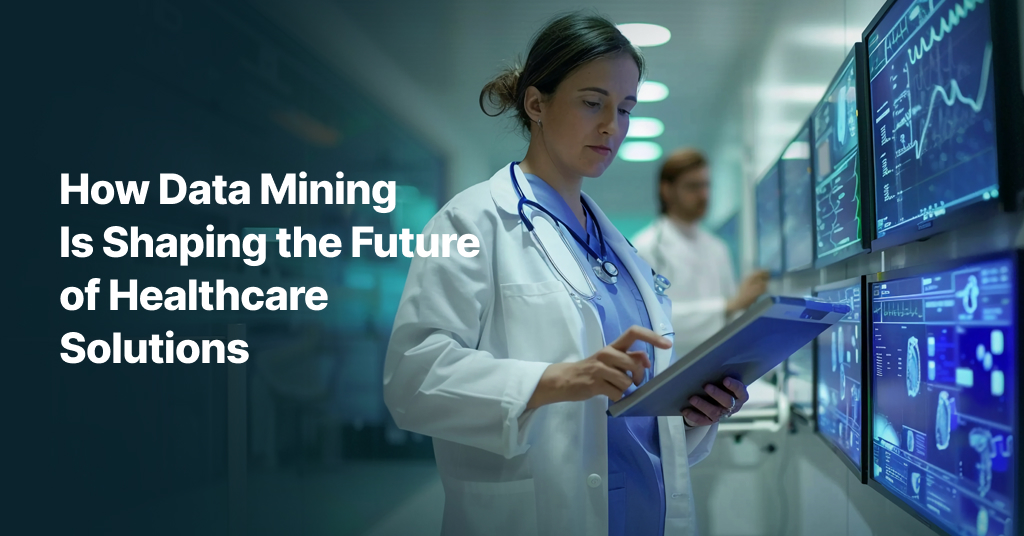
The healthcare sector is evolving rapidly, thanks to advances in artificial intelligence and machine learning. The focus has now shifted from standard treatments to personalized patient care, effective disease diagnostics, and advanced drug research. At the center of this transformation is healthcare data mining.
Today, healthcare providers can collect vast amounts of information through electronic health records, remote patient monitoring tools, and mobile health apps. By analyzing this data, they can uncover valuable insights to discover new frontiers in healthcare research and make informed decisions. Let’s explore how data mining is making a positive shift in healthcare and allowing medical organizations to enhance treatment efficacies and patient outcomes in the modern world.
Table of content
Benefits of Data Mining in Healthcare
With the increasing demand by patients for better, faster, and more personalized services, effective data mining has become a necessity in healthcare. Extracting and analyzing plenty of medical data available in EHRs (electronic health records) and other sources can help healthcare organization in:
- Improved Patient Care and Diagnostic Accuracy
One of the most crucial applications of data mining in healthcare is the accurate diagnosis of critical diseases like cancer. It can help in sifting through vast amounts of genomic data, clinical records, and imaging data to identify, predict, and classify tumor progressions. Also, through data mining, you can analyze data points such as patient demographics, past medical history, genetic information, and lifestyle factors to make improved treatment plans for enhanced patient care.
- Evidence-Based Decision-Making in Clinical Settings
Data mining can also aid in the development of Clinical Disease Support Systems (CDSS) to identify patterns and relationships that may not be immediately apparent to human observers and make informed decisions based on evidence-based knowledge. By applying machine learning algorithms to historical medical records, data mining can predict patient outcomes or the likelihood of developing certain conditions such as sepsis or heart failure. This predictive capability helps CDSS flag high-risk patients and prompt early intervention.
For instance, the University of Pennsylvania Health System implemented a machine learning-based CDSS that significantly improved the early detection of sepsis, leading to better patient outcomes.
- Detection of Insurance Fraud
Insurance fraud in healthcare is one of the prevalent issues, causing over $300B in financial loss every year, as per the last research by NHCAA [Source]. Through data mining techniques and associated tools, a large volume of claim data can be scraped from relevant sources and analyzed to identify unusual patterns and detect outliers. These deviations can help in detecting potential fraudulent activities and prevent or reject fabricated claim submissions.
One of its prominent real-world applications is the MFAD (Medicaid Fraud and Abuse Detection) system used by the Texas Health and Human Services Commission. Utilizing a neural network model and the scraped data from vast sources, this system analyzes unusual claim patterns to identify numerous suspects and has helped the department recover millions of dollars.
- Evaluation of Treatment Efficiency
Data mining algorithms help healthcare providers discover valuable knowledge, such as trends, patterns, characteristics, and anomalies within patient data. This knowledge can be used to conduct comparative studies on patient outcomes across different treatment protocols. By analyzing the results of various treatments, healthcare providers can determine which methods are most effective for specific conditions. Additionally, they can identify any adverse effects or complications associated with particular treatments, enabling prompt adjustments and personalized care.
- Predictive Analytics
Another benefit of data mining in healthcare is that providers can predict which patients are at higher risk for certain illnesses, estimate the likelihood of hospital readmissions, or anticipate disease progression to make informed decisions. By referring to historical patient records, lifestyle factors, and genomic data, healthcare providers can anticipate the risk factors associated with several critical illnesses and intervene proactively.
For example, by analyzing data from diabetic patients, predictive models can identify those at the highest risk for complications, enabling targeted interventions.
- Personalized Patient Care through Segmentation
Data mining can help identify patients with similar characteristics (or genotypes) and categorize them into subgroups for more targeted and personalized treatment approaches. This method, known as Personalized Medicine, enables healthcare providers to make a shift from a one-size-fits-all approach and devise tailored care plans based on each group’s specific needs and risk factors.
- Disease Surveillance and Outbreak Detection
Data mining aids public health officials in detecting, monitoring, and managing the spread of infectious diseases by analyzing extensive epidemiological data. This process involves examining patterns related to symptoms, geographic locations, demographics, and other factors influencing disease transmission.
By providing analytical data on early indicators of an outbreak, data mining enables healthcare providers to implement rapid response and containment efforts for public health protection.
- Drug Discovery and Development
Life sciences companies can conduct clinical trials more effectively for improved drug research and development by analyzing real-world data. Leveraging past clinical trial results, genomic data, and scientific literature, researchers can uncover new biomarkers and explore genetic risk factors. Additionally, real-world evidence helps identify specific patient sub-populations that may benefit more from particular drugs. This information can help them accelerate drug discovery and identify potential new uses for existing drugs.
Key Steps in the Healthcare Data Mining Process
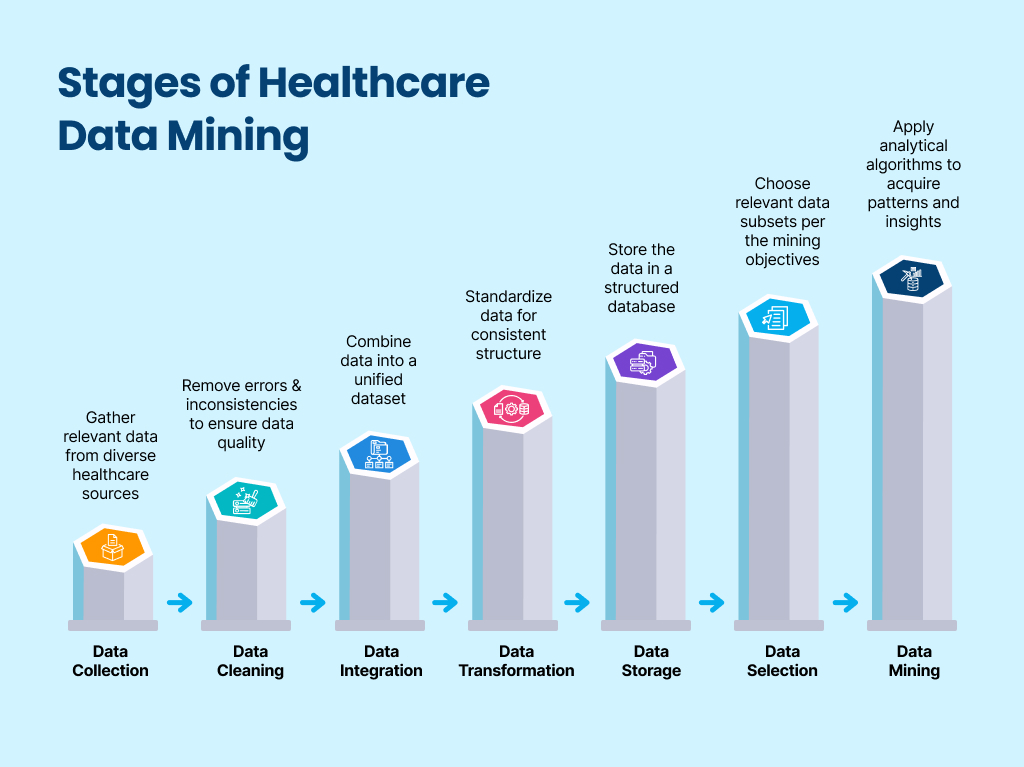
Challenges Involved in Healthcare Data Mining
1. Data Quality (Incomplete/Invalid Details)
For healthcare data mining tools to be effective, the existing or scraped data must be of high quality (free from duplicates, typing errors, and inconsistencies). The challenge arises when the medical data that needs to be analyzed consists of incomplete, obsolete, or invalid details. Analysis based on such data can mislead healthcare providers, impacting their bottom line. To maintain data hygiene, an effective data governance framework is required within an organization. This involves equipping data professionals with the skills and tools necessary for regular data audits and quality control.
2. Data Protection
Patient information is highly sensitive, and any breach or misuse of this data can have severe consequences, including legal penalties, financial losses, and damage to the company’s reputation. Data mining tools, if not properly secured, can expose sensitive information to unauthorized parties, increasing the chances of data breaches. Businesses must invest in robust security measures and data encryption to mitigate these risks and ensure that patient data remains protected against cyber threats.
3. Compliance with Regulations
Regulations such as HIPAA (Health Insurance Portability and Accountability Act). and GDPR (General Data Protection Regulation) imposes strict requirements on how healthcare data is collected, stored, and used. Failure to comply with these regulations can result in hefty penalties and legal issues. Ensuring that data mining practices adhere to these regulations requires continuous monitoring, adaptation to changing laws, and rigorous implementation of data security measures.
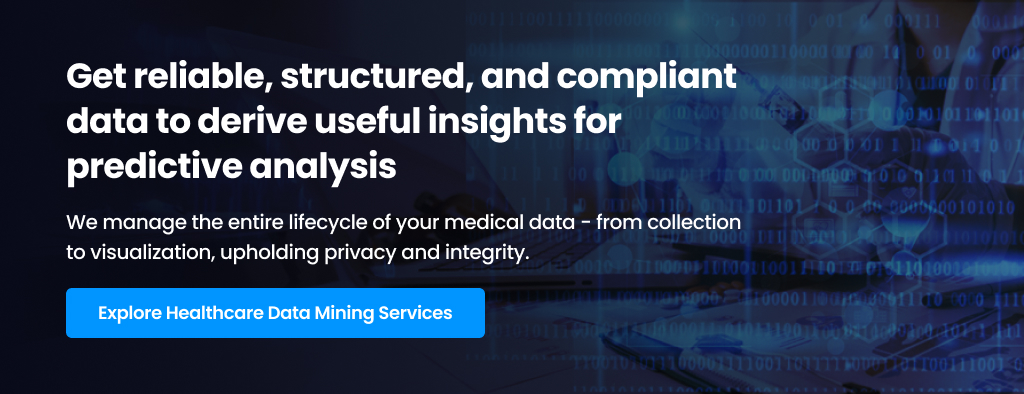
Latest Trends in Healthcare Data Mining
With the latest advancements in various technologies, such as IoT, AI, and ML, the scope of healthcare data mining has also broadened. Let’s understand how.
1. Internet of Medical Things (IoMT)
IoMT is revolutionizing the medical sector by connecting devices like wearable health monitors, remote patient monitoring (RPM) tools, and other smart devices into an integrated network. Wearable devices, such as smartwatches and fitness trackers, continuously collect real-time health data from patients. This wealth of data is invaluable for data mining processes, as it provides a detailed and ongoing view of a patient’s health. By analyzing this data, healthcare providers can detect anomalies early, monitor chronic conditions more effectively, and gain insights into health trends. This enables more accurate and personalized treatment plans, enhancing overall patient care and management.
2. Telecommunications Technologies
Telemedicine and mHealth apps expand the scope and frequency of data collection, providing more touchpoints for patient health information. Through these apps, the data of a wider population (including those in remote or underserved areas) can be easily collected for predictive analysis. These apps allow patients to easily record and share their health information, such as symptoms, medication adherence, and lifestyle factors, directly from their mobile devices.
3. Artificial Intelligence and Machine Learning
AI and ML models are revolutionizing healthcare data mining by automating data collection and analysis. Along with extracting data from relevant/specified sources, these models can organize unstructured information (utilizing NLP technology) and integrate IoT devices for real-time patient monitoring. Additionally, they can swiftly process complex medical records, imaging, and genomic data, uncovering patterns and insights that drive better diagnosis, treatment, and patient outcomes.
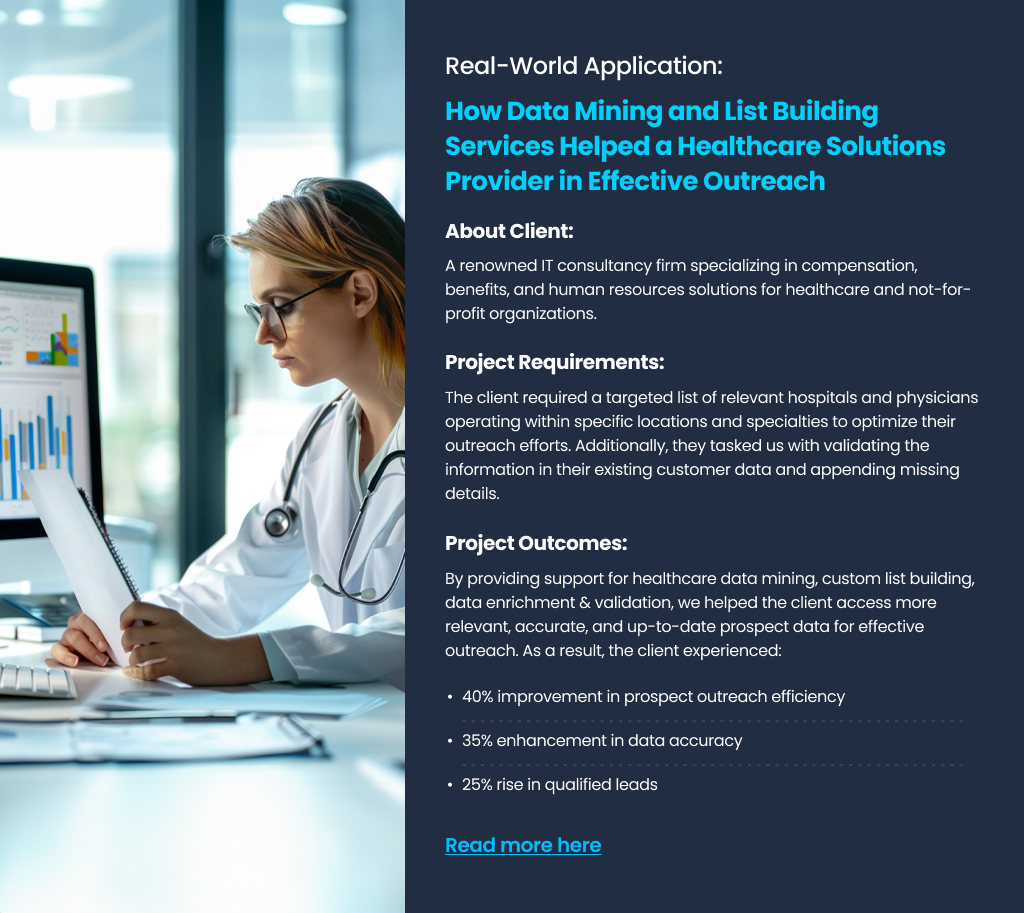
Final Thoughts
Data mining in healthcare serves as a vital catalyst for decision-making and innovation, driving the industry toward more precise, efficient, and personalized patient treatments. Reliable information lies at the heart of evidence-based care, and advancements in AI and ML technologies have significantly expanded the capabilities of data.
The directive is clear for all medical professionals and clinical researchers: Harness healthcare data mining techniques and secure information handling practices to drive improvements in patient care and operational efficiency. Let the data serve as a beacon, guiding us toward a future of healthcare excellence where evidence-driven practices lead to better health outcomes for all.