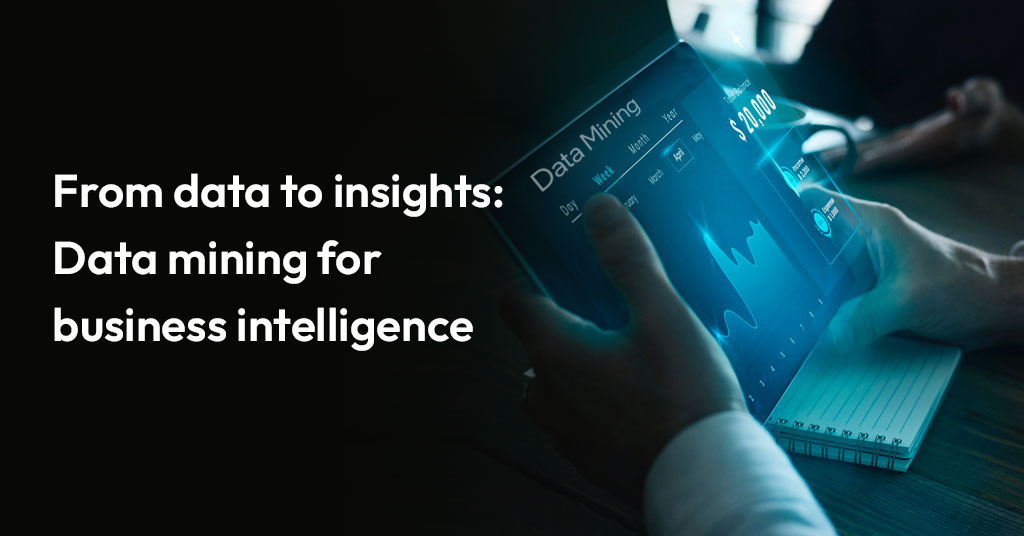
Business data mining has become fundamental to data-driven decision-making. We will discuss data mining for business intelligence as a strategic approach to collecting relevant data. It covers industry-specific applications, the importance of data mining & key strategies and outlines a detailed workflow for internal implementation. The mined data is instrumental in gaining business intelligence as it provides actionable insights into consumer behavior, sales strategies, and overall performance.
The blog also explores professional data mining services as an alternative for enhanced data accuracy and compliance with industry standards. Whether done internally or with professional assistance, data mining maximizes the utility of available data.
Table of content
Introduction
In this era of information abundance, we now have more knowledge at our fingertips than ever before. However, sifting through this data comes with certain challenges in uncovering valuable insights in the sheer volume. This is where data mining comes into play. Leveraging different data collection and scraping techniques allows businesses to gather relevant data and analyze it to identify patterns for better decision-making.
This blog discusses the ins and outs of business data mining, its industry-specific application, and some of the best strategies. We will also explore the option of selecting professional data mining service providers and their benefits over doing it internally. So read on and get a comprehensive view of B2B data mining for business analytics.
What is data mining in business analytics?
Data mining entails collecting heterogeneous data using appropriate API calls, SQL queries, scraping scripts, and web crawlers. This data is then analyzed to discover patterns, correlations, and insights to support decision-making.
In business analytics, this process caters to mining business data (sales, inventory, website traffic, customer interactions, etc) for gaining insights.
Who needs data mining for business intelligence?
Data mining is prominent across multiple industries. Discover how companies are using it:
- Retailers mine B2B data such as sales transactions, brand details, SKUs, product descriptions, reviews, customer demographics, and purchasing history. This data helps them decide an appropriate inventory size, personalize their outreach, and enhance the customer experience.
- Financial institutions (banks, NBFCs, etc) implement data mining for business intelligence. They analyze the collected data (stock prices, financial statements, credit scores, insurance policies, etc) to detect fraudulent activities, assess credit risk, and identify investment opportunities.
- Healthcare institutions leverage business data mining to gather data on medical imaging, historical health records, and patient demographics. Using this data, they can offer personalized treatment plans, identify increasing instances of a particular disease, and issue advisories. Another way data mining is utilized is to compile profiles of healthcare professionals that match specific hiring criteria. Read our success story on Data Mining and Research for Healthcare.
- Educational institutions mine student data such as course enrollment, student preferences, and learning outcomes to identify trends in the education sector. This knowledge enables them to customize curriculum and improve course planning decisions.
Key things to consider when mining B2B data for business analytics
When mining data for business analytics, there are certain things to keep in mind, including:
Define your data mining goals
Understanding your reason for mining B2B data is crucial as it sets the foundation for the entire data mining process. This could be reducing churn, optimizing pricing, or identifying new market opportunities. Additionally, consider how you will use the insights in your decision-making process. Will you utilize them in your sales strategy or strategize for operational improvements?
Decide on a storage and information-sharing channel
Another important thing is to consider how you will store and share the mined B2B data. This involves selecting the appropriate technology infrastructure and platforms to store, process, and share the data and insights generated from data mining.
Evaluate your data mining budget
B2B data mining involves certain costs, such as tools and scrapers, server and storage charges, etc. A thorough analysis of your requirements and the budget will allow you to gauge the feasibility of mining business data in-house or partnering with a data mining service provider.
An internal business data mining workflow
A typical business data mining workflow involves several key steps, from data source identification to data analysis for generating insights. Here’s a breakdown of the workflow:
1. Data source identification
After evaluating your requirements, identify relevant data sources. For example, when mining financial data for business intelligence, you can refer to public data aggregators such as Bloomberg Terminal and S&P Capital IQ. Similarly, if you’re mining internal data for performance analysis, you can source income statements, balance sheets, and financial ratios like profitability, debt-to-equity, etc.
2. Using APIs, custom scripts, web crawlers, or manual techniques to scrape data
Once you’ve identified relevant sources, use available APIs or develop customized scripts to automate data extraction from public databases and websites. Additionally, you can also integrate web crawlers into your website. These bots navigate through web pages or online sources, gathering and extracting data based on predefined criteria.
3. Data compilation
Integrate the data you have compiled from various sources into a centralized repository, such as a data warehouse or a big data platform (e.g., Hadoop or Spark).
4. Data processing and standardization
Process this data to ensure consistency in formats and prepare it for analysis. This involves cleaning data to remove errors or inconsistencies, handling missing values, transforming data into a suitable format, reducing dimensionality if necessary, and converting it into a standardized format (CSV, Excel, MS Word, Text, etc).
5. Data analysis
Once you have a set of clean and consistent data, you can proceed to draw actionable insights. These insights may include market trends, customer behavior patterns, performance metrics, and optimization opportunities.
Some other B2B data mining strategies
Here is a list of a few commonly used B2B data mining strategies:
- API Integration: Integrating with third-party APIs to access and retrieve structured data from platforms such as LinkedIn, Salesforce, and Crunchbase, enabling seamless data extraction.
- Data Aggregation: Aggregating data from multiple sources, such as public records, government databases, and trade publications, to compile comprehensive datasets for analysis.
- Database Purchase: Purchasing proprietary datasets from specialized vendors or data brokers to supplement internal data sources.
- Custom Data Collection: Designing and implementing custom data collection methods, such as online surveys, questionnaires, and data enrichment services, to gather specific information tailored to business needs.
- Data Partnerships: Establishing partnerships with other businesses or organizations to exchange or access data, enabling mutual benefits and expanding data mining opportunities.
The cost of mining data for business intelligence
Implementing business data mining internally is associated with certain cost considerations. Here is a rough breakdown:
● Data mining technologies and infrastructure
This includes the expenses of sourcing custom-written scripts, APIs, licensing fees, one-time purchases, or subscription costs of software and tools used for data mining. Additionally, this also covers the investment in hardware infrastructure, including servers, storage systems, and other computing resources.
● Hiring data mining experts
When managed internally, these expenses extend to salaries and wages for personnel engaged in data mining, encompassing data miners, analysts, and other IT professionals. Moreover, the costs associated with training and retaining these individuals accumulate over time.
● Scaling costs
As the volume of data and the scope of analysis expand, additional resources, such as storage, computing power, and specialized personnel, may be required. Such rising expenses can strain your budget and hinder your ability to scale effectively.
How data mining services can help you reduce these costs?
Partnering with a data mining service provider eliminates additional overhead expenses. As these providers have established setup and workflow for mining business data, you only have to cater to a basic service fee encompassing all costs associated with business data mining.
For complex data mining requirements, such as those involving financial or patient healthcare data, partnering with experienced service providers is often more effective. These providers prioritize data security, integrity, and accuracy throughout the process. Moreover, their expertise ensures that sensitive data is handled with the utmost care and precision.
To give you an estimate, one of our IT consulting and servicing clients in the healthcare industry experienced a substantial boost in the accuracy of their data (35%) due to our diligent data handling, verification, and validation.
Other benefits of a professional service provider
In addition to optimizing the costs associated with business data mining, a professional service provider offers further benefits, such as:
1. Industry-relevant expertise and experience
Professional service providers have a team of experts possessing knowledge of industry-specific data sources, compliance regulations (such as HIPAA for healthcare data), and best practices. Additionally, as the future calls for AI-driven data mining, they can effectively incorporate advanced machine learning algorithms to enhance the efficiency and accuracy of your process.
2. Enhanced data acquisition speed
B2B Data mining service providers have expertise in integrating data from disparate sources, including databases, APIs, web scraping, and sensor networks. Additionally, some data mining services leverage real-time data streaming technologies to acquire data as it is generated. Their proactiveness allows you to experience remarkable data acquisition speeds. For instance, one of our clients experienced up to 5X faster acquisition of ESG (Environmental, Social, and Governance) data.
3. Established technology infrastructure
Partnering with a professional service provider allows you to access an established technology infrastructure and cutting-edge tools. This includes hardware, software, and large-scale data management systems such as Amazon RDS, Apache Cassandra, and MongoDB.
4. Customized data mining solutions
One of the key benefits of partnering with a data mining service provider is the high level of customization they offer. With years of experience, professional service providers can quickly implement precise solutions tailored to address your specific challenges. This expertise allows them to adapt their services to meet your unique needs.
5. Scalability
Professional data mining services are designed to handle large volumes of data efficiently. As businesses grow and accumulate more data, these service providers can scale up their infrastructure and resources to cope with the increased processing demands. Additionally, they can incorporate new data sources, adjust processing models, and iterate on strategies based on your feedback.
What should you look for in a data mining service provider?
Opting for professional B2B data mining services is advantageous for sure, however, you must consider a few things before finalizing a service provider. Here are some of them:
- Technological Capabilities: Ensure the provider has access to advanced tools (such as Apache Spark, Python Libraries, Hadoop, etc), technologies, and analytical methods required for your specific data mining needs.
- Experience and Expertise: Look for a provider with a proven track record and expertise in data mining for business analytics. Check their experience in your industry and the types of projects they’ve successfully handled.
- Pricing Model: When assessing a provider’s pricing structure, make sure they offer various engagement models, such as pay-per-use and subscriptions. Also, select those who can tailor a service package to your specific requirements.
- Turnaround Time: Faster turnaround times allow you to access consolidated business data and expedite your analysis. This enables you to draw actionable insights and expedite decision-making.
- Communication and Collaboration: Look for a provider that fosters open communication and keeps you informed and involved throughout the data mining process.
- Data Security Measures: Make sure the data mining service provider has stringent security protocols to safeguard your data. They must also adhere to data privacy regulations such as GDPR, CCPA, etc. This minimizes the risk of inappropriate customer data usage and potential security breaches.
The final verdict
Sourcing and analyzing B2B data allows you to gather invaluable insights for informed decision-making and strategic business planning. However, ensuring compliance with data security and privacy regulations while collecting business data is crucial; and that’s where a lot of companies struggle. One efficient way to sidestep this challenge is opting for professional business data mining services. These service providers implement robust security measures to safeguard sensitive information while ensuring maximum accuracy. So collaborate with a data mining service provider to benefit from highly accurate data and compliant mining services.
Frequently Asked Questions
1. What is the difference between data mining and data analytics?
While the terms are often used synonymously, they are distinct concepts. Data mining primarily focuses on the process of collecting, processing, validating, and collating comprehensive datasets that are used for discovering patterns, correlations, and insights. On the other hand, data analytics is the broader process of analyzing, interpreting, and visualizing data, making data mining a subset of data analytics.
2. Why is data mining challenging?
Data mining poses several challenges due to various factors:
- Data Quality: Incomplete, inconsistent, or erroneous data leads to incorrect conclusions, making it crucial to ensure data quality before analysis.
- Data Privacy and Security: Businesses must follow best practices and comply with regulations to prevent sensitive data from being leaked or hacked, adding complexity to data mining processes.
- Volume of Data: The volume of data being generated can be overwhelming, leading to longer processing times and higher costs,
- Interpretation of Results: Interpreting data mining results can be challenging as patterns and relationships may not be immediately clear, requiring further analysis and domain expertise.
3. What are some commonly used data mining techniques?
Here is a list of widely used data mining techniques:
- Classification: Categorizing data into predefined classes or labels based on input variables.
- Clustering: Grouping similar data points into clusters to identify patterns or structures.
- Regression Analysis: Modeling the relationship between dependent and independent variables to predict continuous outcomes.
- Text Mining: Extracting valuable insights from unstructured text data, such as sentiment analysis or topic modeling.
- Feature Selection: Identifying and selecting the most relevant features or variables for predictive modeling.
- Dimensionality Reduction: Reducing the number of features in a dataset while preserving important information to improve model efficiency and interpretability.
Association Rules: Identifying relationships or associations between variables in large datasets to uncover patterns or dependencies.